Combining human and machine learning for morphological analysis of galaxy images
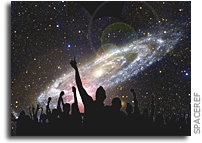
Evan Kuminski, Joe George, John Wallin, Lior Shamir
(Submitted on 28 Sep 2014)
The increasing importance of digital sky surveys collecting many millions of galaxy images has reinforced the need for robust methods that can perform morphological analysis of large galaxy image databases. Citizen science initiatives such as Galaxy Zoo showed that large datasets of galaxy images can be analyzed effectively by non-scientist volunteers, but since databases generated by robotic telescopes grow much faster than the processing power of any group of citizen scientists, it is clear that computer analysis is required. Here we propose to use citizen science data for training machine learning systems, and show experimental results demonstrating that machine learning systems can be trained with citizen science data. Our findings show that the performance of machine learning depends on the quality of the data, which can be improved by using samples that have a high degree of agreement between the citizen scientists. The source code of the method is publicly available.
Comments:
PASP, accepted
Subjects:
Instrumentation and Methods for Astrophysics (astro-ph.IM); Astrophysics of Galaxies (astro-ph.GA); Computer Vision and Pattern Recognition (cs.CV); Learning (cs.LG)
Cite as:
arXiv:1409.7935 [astro-ph.IM] (or arXiv:1409.7935v1 [astro-ph.IM] for this version)
Submission history
From: Lior Shamir
[v1] Sun, 28 Sep 2014 17:47:35 GMT (184kb)
http://arxiv.org/abs/1409.7935