Discovering Long-period Exoplanets Using Deep Learning With Citizen Science Labels
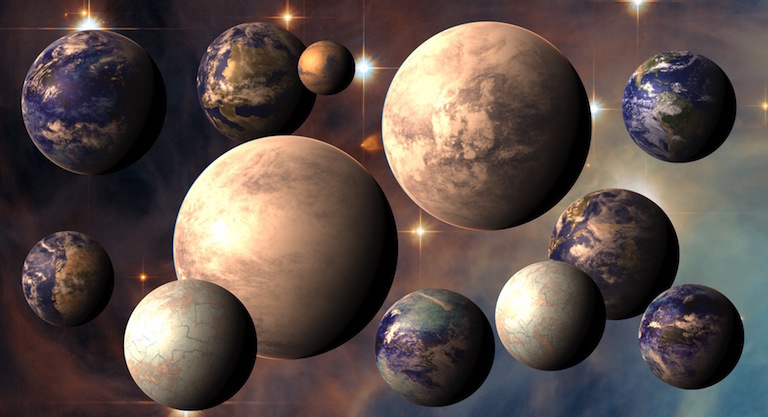
Automated planetary transit detection has become vital to prioritize candidates for expert analysis given the scale of modern telescopic surveys.
While current methods for short-period exoplanet detection work effectively due to periodicity in the light curves, there lacks a robust approach for detecting single-transit events. However, volunteer-labelled transits recently collected by the Planet Hunters TESS (PHT) project now provide an unprecedented opportunity to investigate a data-driven approach to long-period exoplanet detection.
In this work, we train a 1-D convolutional neural network to classify planetary transits using PHT volunteer scores as training data. We find using volunteer scores significantly improves performance over synthetic data, and enables the recovery of known planets at a precision and rate matching that of the volunteers. Importantly, the model also recovers transits found by volunteers but missed by current automated methods.
Shreshth A. Malik, Nora L. Eisner, Chris J. Lintott, Yarin Gal
Comments: Accepted at the Machine Learning and the Physical Sciences workshop, NeurIPS 2022
Subjects: Earth and Planetary Astrophysics (astro-ph.EP); Instrumentation and Methods for Astrophysics (astro-ph.IM); Machine Learning (cs.LG)
Cite as: arXiv:2211.06903 [astro-ph.EP] (or arXiv:2211.06903v1 [astro-ph.EP] for this version)
Submission history
From: Shreshth Malik
[v1] Sun, 13 Nov 2022 13:33:34 UTC (3,341 KB)
https://arxiv.org/abs/2211.06903